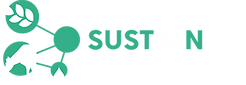
Our 2024 Studentships
We are delighted to announce our fully-funded, four-year PhD studentships in the application of Artificial Intelligence to sustainable agri-food, to start in October 2024.
Explore the projects in more detail below.

Integrated Analyses to Promote Sustainable Soil Health (SUSTAINable Soils)
Prof Mat Goddard, University of Lincoln; Dr Nicolas Rubido, University of Aberdeen; Prof Simon Parsons, University of Lincoln; Dr Dan Magnone, University of Lincoln
Soils produce 97% of the world’s food calories; provide key water regulation processes for flood management; harbour the highest levels of biodiversity on Earth; and contain the largest dynamic reservoir of carbon on Earth. However, there is virtually no integrated holistic knowledge of soil properties, and more importantly how these are affected by land use and management change. This project will contribute to an evidence base that will support land-use change decisions to help mitigate climate change, with opportunity to work across a broad range of computer science, applied mathematics, and environmental and soil science areas. Read more.

Service Robots Interventions Promoting More Sustainable Buyers’ Choices
in Supermarkets
Dr Francesco Del Duchetto, University of Lincoln; Dr David McBey, University of Aberdeen; Dr Leonardo Guevara, University of Lincoln
Simple dietary changes by individuals and households can have a great effect in improving human health and curtailing greenhouse gas emissions. This can be achieved, for example, by encouraging the consumption of more plant-based foods through a process called “nudging”, which entails performing interventions to alter people’s behaviour in a predictable way.
This project will explore the effectiveness of automating the provision of nudges with AI and service robotics in food retail locations. If effective, this approach could be adopted by supermarkets and restaurants where we can expect robots will be widely deployed in the future as customer assistants. Read more

Flavour-centric Innovation for Cultivated Meat: Bridging the Sensory Gap in Cellular Agriculture
Dr Tassos Koidis, Queen's University Belfast; Prof Georgios Leontidis, University of Aberdeen; Multus Biotechnology
As the global population rapidly increases, the quest for sustainable and nutritious alternatives to traditional animal products becomes crucial. Cellular agriculture, which involves growing animal cells in a controlled environment to produce food, offers a promising solution. However, the success of cultivated meat in the market hinges on replicating the sensory experiences of traditional meat. This project aims to transform cellular agriculture by prioritizing flavour-centric production while maintaining viable manufacturing volumes. Read more.
Available

Food Authentication Using Portable Sensors: Addressing Food Fraud and Food Mislabelling
Prof Hui Wang, Queen's University Belfast; Prof Louise Manning, University of Lincoln
Worried about food fraud and mislabelling? These issues erode consumer confidence in the agri-food system, leaving us questioning the authenticity of what we eat. This project proposes an innovative solution: a portable food authenticator that leverages everyday technology to rebuild trust. Using portable sensors and machine learning, sophisticated algorithms trained on real data will identify food types, detect adulteration, and even uncover discrepancies between labelled information and the actual food composition. Read more.
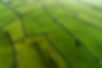

Artificial Intelligence for Satellite Assessment in Grassland Ecosystems
(AI-SAGE)
Dr Robert Atkinson, University of Strathclyde; Dr Lan Qie, University of Lincoln; Dr Christos Tachtatzis, University of Strathclyde; Lactalis
Satellite Earth Observation (EO) Data has emerged as a game-changer in accurately assessing plant biodiversity and ecosystem functions within agricultural landscapes, spanning both grassland and arable terrains. The current challenges in harnessing EO data through Deep Neural Networks (DNNs) is not a scarcity of data, but the limited availability of detailed, pixel-level annotations. The studentship aims to: extract biodiversity indicators in grassland landscapes;
bridge this gap between large volumes of data and limited pixel level annotations; explore domain shift (EO trained models typically do not transfer between geographies); and develop guidance on grassland management strategies. Read more.

Understanding Multi-Objective Optimisation Algorithms for Land Use
Dr Daniel Magnone, University of Lincoln; Professor Nir Oren, University of Aberdeen; Professor Simon Parsons, University of Lincoln
The UK’s land use can be considered a complex, multi-objective optimisation problem, balancing economic yield, biodiversity enhancement, and carbon storage. With the recent introduction of the Environmental Land Management Scheme (ELMS), the need for a decision- support system to help stakeholders understand the impacts and trade-offs of land parcel allocation is clear and timely. This PhD project will utilise advances in formal argumentation theory and dialogue to encode, reason, and explain the behaviour of multi-objective optimisation algorithms. The research will focus on optimising carbon storage, biodiversity enhancement, and economic yield from agriculture. Read more.

Machine Learning and Bayesian Modelling Approaches for Big Data in Beef Agriculture & Food Production
Dr Arran Hodgkinson, Queen's University Belfast; Prof Ilias Kyriazakis, Queen’s University Belfast; Dr Mingjun Zhong, University of Aberdeen; Agri-Food and Biosciences Institute
Machine learning (ML) is an invaluable modern technique whose insights could radically change farming practices, both to the benefit of livestock and farmers. As such, we are looking for a motivated candidate with a strong background in data analytics, statistics, and machine learning to take on this exciting project. Fundamentally, this project intends to utilise cutting-edge ML and probability-based statistical strategies to directly address inefficiencies in feeding practices within the bovine livestock supply chain, employing modelling techniques alongside real-world databases. These models will seek to increase sustainability and animal welfare, by facilitating an individual- or subpopulation-based approach to feeding and nutritional consideration. Read more.

Novel Applications of Artificial Intelligence Technologies in Veterinary Ultrasound Scanners
Christos Tachtatzis, University of Strathclyde; Prof Sam Martin, University of Aberdeen; Fraser Hamilton, IMV Imaging (UK) Ltd (Industry Supervisor)
Fish production for human consumption is increasing globally to meet food demands from a growing population. Interventions must be introduced to de-risk the impact of climate change on the growing aquaculture industry. This exciting PhD aims to improve sustainability in the aquaculture industry through the combination of ultrasound and AI. The goal is to monitor fish health, reduce the risk of disease and improve efficiency in the industry. Read more.

Towards Sustainable Net-Zero Farming Using Machine Learning
Dr Vladimir Stankovic, University of Strathclyde; Dr Marta Dondini, University of Aberdeen; Dr Lina Stankovic, University of Strathclyde; RHD (Scotland) Ltd
The agri-sector, especially dairy farming, is a major contributor to greenhouse gas (GHG) emissions. Understanding the variation in GHG emissions among different farming practices helps to identify sustainable approaches that reduce pollution. The aim of the PhD research is to develop an AI-driven methodology for holistic quantification of GHG emissions in dairy farming, taking into account all sources of GHG emissions and provide recommendations for GHG reduction. Besides hands-on mentored research, the student will develop broader research skills related to AI and mathematical modelling, and transferrable skills related to professional development. Read more.

Development of a milk (mid-infrared) MIR Spectral deep learning neural network as a classifier model for GHG emission profiles in dairy cattle
Prof Craig Michie, University of Strathclyde; Prof Chris Creevey, Queen’s University Belfast; Dr Mazdak Salavati, SRUC
A recent technology which has been taken up globally is milk mid-infrared spectroscopy or MIR. The project aims to develop an MIR spectral deep learning neural network as a classifier model for Green House Gas (GHG) emission profiles in dairy cattle – harnessing the power of digital transformation and field applications of AI to tackle the urgent issue of reducing the carbon footprint of the dairy supply chain. Read more.